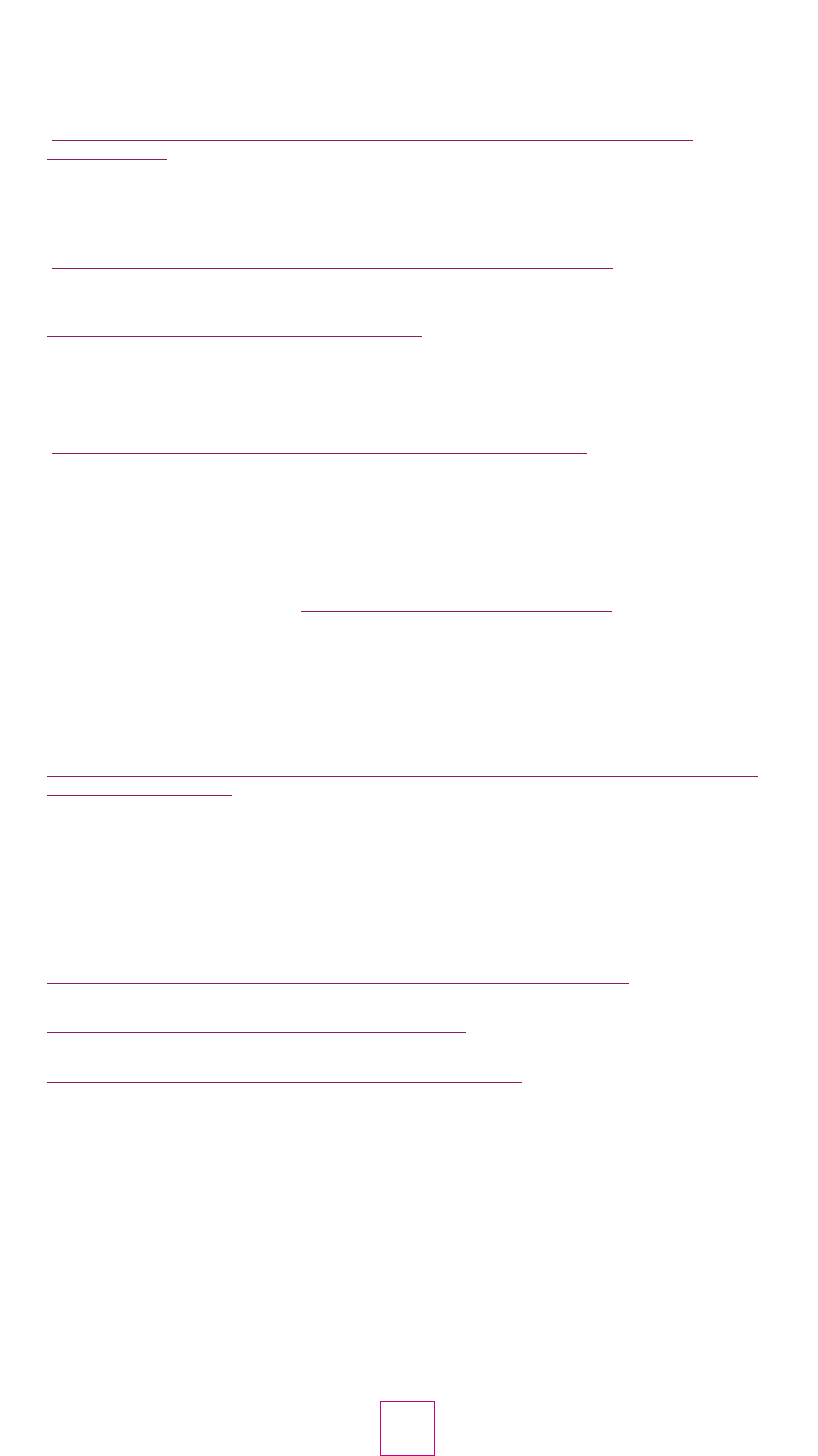
44
Nezhyvenko, O. (2018), ‘Methodology Behind the Measurement of the Platform Economy (Survey on the Plat-
form Economy in Ukraine)’, presentation for Expert workshop 'Measuring the platform economy: size and
impact', CEPS, Brussels
(
http://www.inclusivegrowth.eu/files/Call-5/INGRID2_ExpertWorkshop_Session1_Oksana-
Nezhyvenko.pdf).
Nielsen (2014), ‘Global share community report: Is sharing the new buying?’, Nielsen.com.
Office for National Statistics (2017), ‘Internet access—households and individuals’, Statistical Bull.
ORB International (2017), ‘Uber poll 2017 - Flexibility key for drivers’, ORB International, September
(http://www.orb-international.com/2017/09/26/uber-poll-flexibility-key-drivers
).
ORB International (2018), ‘New ORB International poll for Uber and Oxford Martin School – Uber happy? Work
and well-being in the ‘gig economy’’, 2 October, available at
www.orbinternational.com/2018/10/02/uberhappy
.
Owyang, J., Samuel, A. and Grenville, A. (2014), ‘Sharing is the new buying, how to win in the collaborative
economy’, Vision Critical and Crowd companies.
Pesole, A. et al. (2018), ‘Platform Workers in Europe Evidence from the COLLEEM Survey’, EUR - Scientific and
Technical Research Reports, Publications Office of the European Union
(http://publications.jrc.ec.europa.eu/repository/handle/111111111/52393
).
PwC (2015), ‘The sharing economy’, Consumer Intelligence Series, PricewaterhouseCoopers.
PWC (2018), ‘Share Economy 2017: The New Business Model’, PricewaterhouseCoopers.
Riso, S. (2019), ‘Mapping the contours of the platform economy’, Working Paper, Eurofound, Dublin.
Robles, B.J. and M. McGee (2016), ‘Exploring Online and Offline Informal Work: Findings from the Enterprising
and Informal Work Activities (EIWA) Survey’, SSRN Scholarly Paper ID 2875204, Social Science Research
Network, Rochester, NY, October (https://papers.ssrn.com/abstract=2875204
).
Russel, M. and M. Klassen (2019), Mining the Social Web: Data Mining Facebook, Twitter, LinkedIn, Instagram,
GitHub, and More, O’Reilly Media, Inc.
Schmidt, F.A. (2017), ‘Digital Labour Markets in the Platform Economy: Mapping the Political Challenges of
Crowd Work and Gig Work’.
Serfling, O. (2018), ‘Crowdworking Monitor Nr. 1’, Für das Verbundprojekt „Crowdworking Monitor’. CIVEY, Hoch-
schule Rhein-Waal, gefördert durch Bundesministerium für Arbeit und Soziales. Abgerufen von
https://www.bmas.de/DE/Presse/Meldungen/2018/ausmass-von-plattformarbeit-in-deutschland-
hoeher-als-erwartet. html.
Silberman, M.S. (2017), ‘Fifteen criteria for a fairer gig economy’, democratization, Vol. 61, No. 4, pp. 589–622.
Smith, A. (2016a), ‘Shared, collaborative and on demand: The new digital economy’, Pew Research Center.
Smith, A. (2016b), ‘Gig Work, Online Selling and Home Sharing’, Pew Research Center.
Statens Offentliga Utredningar (SOU) (2017), ‘Ett arbetsliv I förändring – hur påverkas ansvaret för arbetsmiljön?’,
Stockholm.
Statistics Canada (2017), ‘The sharing economy in Canada’, Press release, 28 February, available at
https://www150.statcan.gc.ca/n1/daily-quotidien/170228/dq170228b-eng.htm
Statistics Canada (2018), ‘Digital economy, July 2017 to June 2018’, Press release, 29 August, available at
https://www.statcan.gc.ca/eng/about/smr09/smr09_094
Statistics Finland (2017), ‘Labour force survey 2017: platform jobs’, available at
http://www.stat.fi/til/tyti/2017/14/tyti_2017_14_2018-04-17_en.pdf
.
Stokes, K., Clarence E., Anderson, L. and Rinne, A. (2014), ‘Making Sense of the UK Collaborative Economy’,
Nesta, London.
Teubner, T. et al. (2017), ‘Price determinants on AirBnB: How reputation pays off in the sharing economy.’,
Journal of Self-Governance & Management Economics, Vol. 5, No. 4, pp. 53–80.
US Bureau of Labor Statistics (2018), Electronically Mediated Work: New Questions in the Contingent Worker
Supplement. Monthly Labor Review, US Bureau of Labor Statistics Washington DC.
vanden Broucke, S. and B. Baesens (2018), Practical Web Scraping for Data Science Best Practices and Exam-
ples with Python, KU Leuven.
Vaughan, R. and Daverio, R. (2016), ‘Assessing the size and presence of the collaborative economy in Europe’,
PwC, London, UK.
Weel, B., Werff, van der, S., Bennaars, H., Scholte, R., Fijnje, J., Westerveld, M. and Mertens, T. (2018), ‘De opkomst
en groei van de kluseconomie in Nederland’, SEO (SEO Economisch Onderzoek), Amsterdam.